Cell-based medicinal products (CBMPs) offer promising opportunities for the treatment of diseases with previously limited or no therapeutic options. However, the complexity and intrinsically fragile nature of these CBMPs create significant challenges in formulation development, analytical characterization, manufacturing, and stability assessment.
This recent study used a FlowCam, Flow Imaging Microscope (FIM) assisted by machine learning software, to measure cell viability and concentration, and to quantify debris particulates. These particulates were the result of forced degradation studies that mimic conditions from cell procurement to administration of product. In particular, the effects of freeze-thawing and shaking on CBMPs were studied.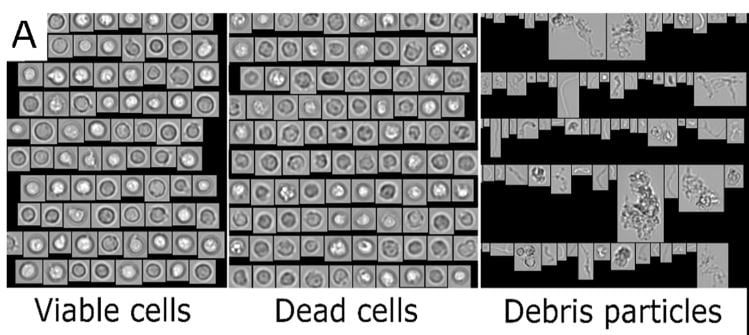
Extensive testing of CBMPs prior to patient administration is a regulatory obligation to assure safety and efficacy of products. Reliable analytical methods for characterization of particles to determine product quality and stability are necessary. In this study a FlowCam 8100 (FIM) was combined with convolutional neural networks (FIM-CNN) to establish a high-throughput method to quantify viable and dead cells, and debris particles in the size range of 1-50 µm.
Given the nature of cells, it can be challenging to fulfill certain particle testing requirements. It was noted that light obscuration, one of the standard pharmacopeial methods for quantification of subvisible particle impurities, is not capable of discriminating between cells and other particulates (due to size, shape and opacity). High-throughput microscopy methods that can provide morphological data are promising tools to evaluate particulates in cell-based products. With the combination of FIM and CNN, reliable concentration data for debris particles and viable cells was obtained.